By Majid Farhadloo and Shashi Shekhar, Department of Computer Science, University of Minnesota
Imagine having a virtual turf guru right at your fingertips, a master of agronomy who understands your turfgrass and lawn needs. With the power of deep learning, artificial intelligence (AI) language models like ChatGPT by OpenAI, Google’s Bard, and LLaMA by Meta, have been making headlines lately with their promising ability to process and understand human language. Imagine if these AI models could become the green thumbs we need to nurture our lawns and greens. Could they serve as virtual lawn care experts, assisting us in buying or growing resilient turfgrass? Might they help us select the grass mixture that will thrive in our local climate?
Picture every query and question you've ever asked on the internet or every article you've read about improving and maintaining your lawn. These AI language models are created by digesting this vast amount of internet information. While they are continuously being improved, they can potentially aid lawn enthusiasts by offering personalized guidelines and recommendations for various tasks. With their data-driven insights, you may be just a few taps or voice commands away from knowing the right amount of water for your lawn or optimizing irrigation schedules. These AI language models may become like the Siri or Alexa of turfgrass! So, do these language models actually offer a user-friendly interface for your turfgrass management needs? The answer is yes and no.
Yes, the available language models could be a helpful question-and-answer tool for turfgrass managers, including homeowners. They can provide prompt responses and guidance by drawing from a pool of internet content to offer hundreds to thousands of lawn care tips. However, it’s important to remember that these models rely on internet data, and their recommendations may not consistently align with your specific needs. For example, a language model might suggest using a particular type of fertilizer based on common recommendations found online; however, this recommendation may not consider the specific nutrient needs of your soil, environmental factors, and the climate conditions in your region. Moreover, these models cannot adapt to the specific conditions at your site, especially when dealing with evolving turfgrass issues, resulting in one-size-fits-all recommendations. Thus, while AI language models can offer some insights, they should be used as a supplementary resource rather than a replacement for expert advice.
Geo-Object detection
What we can do in combination with language models, is harness the power of another valuable tool: geospatial AI (GeoAI). GeoAI is a generalization of traditional AI that addresses unique challenges posed by geospatial data. Its techniques can help us identify turf stress hotspots and uncover areas where disease and certain environmental conditions co-occur.
Last winter, Minnesota experienced severe weather conditions, including frequent melting and freezing along with record-breaking snowfalls, including the Twin Cities witnessing one of the top 15 snowfalls since 1884. As a result, many homes and landscapes suffered from winter stress damage, especially damage from snow mold, a disease caused by a fungus that thrives under snow cover and affects cool-season grasses like those common in Minnesota. Once the snow melts, snow mold can create patches of unhealthy grass and allow weeds to grow and compete for resources and nutrients, leaving an unsightly lawn.
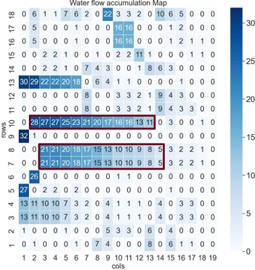
Here's where GeoAI techniques, such as geo-object detection, can be instrumental in analyzing spatial and visual information from a lawn, including climatic conditions, soil type, garden type, elevation, and more. As shown in Figure 1, a water accumulation map that highlights landscape depression could help in identifying areas of potential winter damage in a lawn. These risk zones may be formed due to low-lying spots or poor drainage, and are more prone to have higher moisture levels, making them more susceptible to issues like ice formation and snow mold growth. By utilizing this year-round data, geo-object detection could aid a better understanding of when a lawn might be at risk of damaging disease. It’s exciting to imagine retraining ChatGPT and the like with this rich new source of spatial, visual, and written information, enabling these models to recommend targeted actions, like specific management actions to reduce the incidence of snow mold disease. For further insights on leveraging spatial data, you can refer to our previous article, Fine-grain spatial information may enhance the value of turfgrass cultivar data.
This blog post highlights just a few of the possibilities for using large language models and geo-object detection for assisting turfgrass managers, including homeowners, in their lawn care needs. There are, however, still many uncertainties and challenges that need to be addressed before these techniques can be deployed for end-user applications such as those described above. While language models and geo-object detection techniques can provide recommendations and insights, it may not always be clear how they arrived at those conclusions. This lack of interpretability can make it difficult for users to understand and trust the recommendations. Moreover, the effectiveness of language models and geo-object detection techniques relies heavily on the quality and accuracy of the data used to train them. If the data is incomplete, biased, or inaccurate, it can lead to incorrect recommendations and insights. Advancements in AI have made many things possible, and someday they may be your go-to for lawn care advice.
References
- Brown, Tom, et al. "Language models are few-shot learners." Advances in neural information processing systems 33 (2020): 1877-1901.
- Alba, D. and J. Love (February 6, 2023). "Google releases ChatGPT rival AI 'Bard' to early testers". Los Angeles Times. ISSN 0458-3035. Archived from the original on February 6, 2023.
- Nieva, R. (February 6, 2023). "Google Debuts A ChatGPT Rival Called Bard In Limited Release". Forbes. Archived from the original on February 7, 2023.
- Touvron, Hugo, et al. "Llama: Open and efficient foundation language models." arXiv preprint arXiv:2302.13971 (2023).
- Shekhar, S., and P. Vold. Spatial computing. MIT Press, 2020.
Top 25 snowfalls in the Twin Cities: 1884-2023 (2023) Minnesota Department of Natural Resources. Available at: https://www.dnr.state.mn.us/climate/journal/top_twenty_snowfalls.html (Accessed: 24 July 2023). - Farhadloo, M., and Shekhar, S. (2022, May 24). Fine-grain spatial information may enhance the value of turfgrass cultivar data. https://lowinputturf.umn.edu/fine-grain-spatial-information-may-enhance-value-turfgrass-cultivar-data