By Ce Yang and Alireza Sanaeifar, University of Minnesota
Golf courses are often subject to harsh winter conditions that can result in extensive damage, affecting turf health and overall playability. To combat these challenges, modern technology has come to the rescue. Drone remote sensing has emerged as a powerful tool for collecting high-resolution data, enabling golf course managers to monitor and assess winter damage efficiently. This project, which is part of the WinterTurf project, delves into the use of drone remote sensing data collection on multiple golf courses in both Minnesota and Oregon to detect winter damage. The project employs a combination of hyperspectral and multispectral imagery, and the results are paving the way for early detection and proactive maintenance strategies.
Winter damage detection in Minnesota golf courses
In the past two years, drone-mounted hyperspectral sensors that detect a wide spectrum of light were deployed to capture monthly imagery during the late fall to early winter period and again from March to May. The hyperspectral imagery covered a broad spectral range between 400 nm to 1000 nm, providing detailed information about turfgrass. The data collected during these periods could help assess the extent and severity of winter damage, leading to prompt and targeted turf recovery efforts. The preprocessed hyperspectral images after calibration and georectification were shared with the project team, allowing them to collaboratively analyze and strategize for optimal course management.
Multispectral imaging for winter damage detection in Oregon
Four Oregon golf courses were surveyed monthly using drone multispectral imagery with RGB (red, green and blue), red edge, and two near-infrared bands. This comprehensive dataset was collected in 2022 and 2023, providing an invaluable baseline for comparison and trend analysis. To investigate early winter damage detection, a set of 30 image sets collected by the Oregon team in February and March of both 2022 and 2023 was chosen with diverse topography, winter damage patterns and progression in four golf courses. The use of multispectral imaging allowed the team to assess various aspects of vegetation health and vigor (Figure 1), enabling them to identify subtle signs of winter damage in March or even February, well before they escalate into more significant management issues. As with the Minnesota data, the images were processed, stitched, and georectified. Data was shared with the project team for in-depth examination and further annotation of the winter-damaged areas.
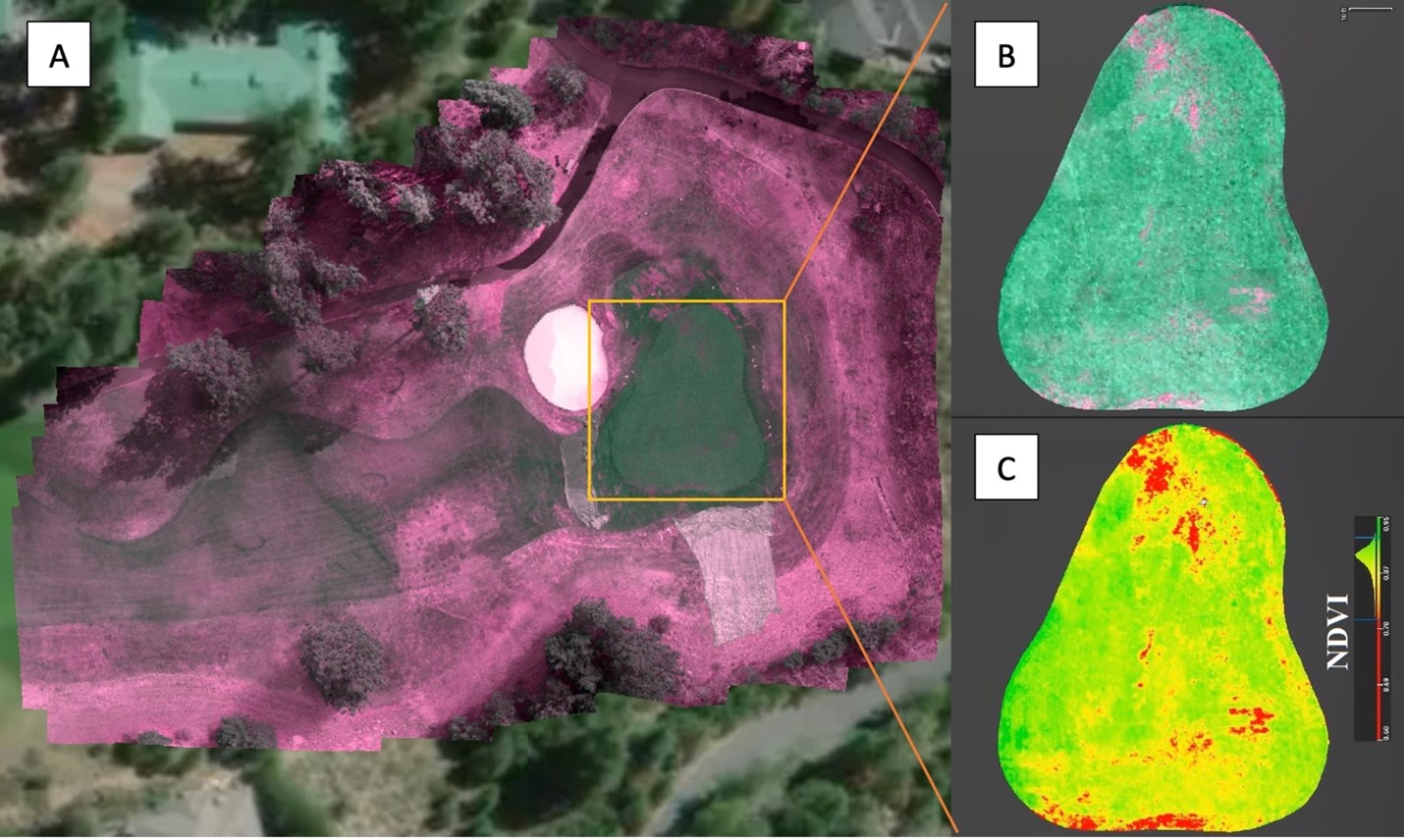
Research progress and future plans
The team currently focuses on image annotation of drone multispectral images to precisely identify and map the winter-damaged areas on the golf courses and use it as ground truth for winter damage detection modeling. The collected data and analysis will serve as a valuable resource for enhancing golf course management practices, providing insights into winter damage patterns and facilitating the development of preventive measures. By combining the strengths of spectral imagery and machine learning modeling, this drone remote sensing initiative will show its potential of aiding the golf course industry to achieve sustainable and visually appealing courses year-round.
In conclusion, the project's use of drone remote sensing on golf courses in Minnesota and Oregon for winter damage detection will showcase the immense potential of this technology. With hyperspectral and multispectral imaging techniques, golf course managers and researchers will gain valuable insights into turf health, enabling proactive decision-making and targeted maintenance efforts. As winter damage annotation progresses followed by detection modeling, the project will contribute to the advancement of golf course management practices, offering a comprehensive approach to tackle the challenges posed by harsh winter conditions.